Setting up local Spark Cluster
EMR presents a convenient method for rapidly deploying a cluster. However, we frequently require an ability to explore locally stored data rapidly. This guide walktlhoughts setting up a minimal local spark cluster fit for stated purpose.
The completed project can be found here.
- PySpark
- Jupyter Notebook connecting to Pyspark
- Writing Iceberg Table Locally
- Writing Iceberg Table to AWS (TBD)
- Reading Parquet files from AWS (TBD)
PySpark
Install Java and Sparks via SDK Man.
$ curl -s "https://get.sdkman.io" | bash
$ sdk install java 17.0.13-zulu
$ sdk install spark 3.5.3
# Uses (sdk list java) OR (sdk list sparks) to see all available version
Install UV
# On macOS and Linux.
curl -LsSf https://astral.sh/uv/install.sh | sh
Jupyter Notebook
Using uv to setup the project and add libraries
$ uv init
$ uv add pyspark jupyterlab
Then we can run bringup the notebook using this command
$ uv run jupyter lab
Minimally we can connect to by creating Sparksession using
from pyspark.sql import SparkSession
spark = SparkSession.builder \
.master("local[*]") \
.appName("spark-demo") \
.config(map={
## Configuration goes here
}) \
.getOrCreate()
Starting spark this way would create a WebUI at 4040. we can inspect this address by evaluating
spark.sparkContext.uiWebUrl
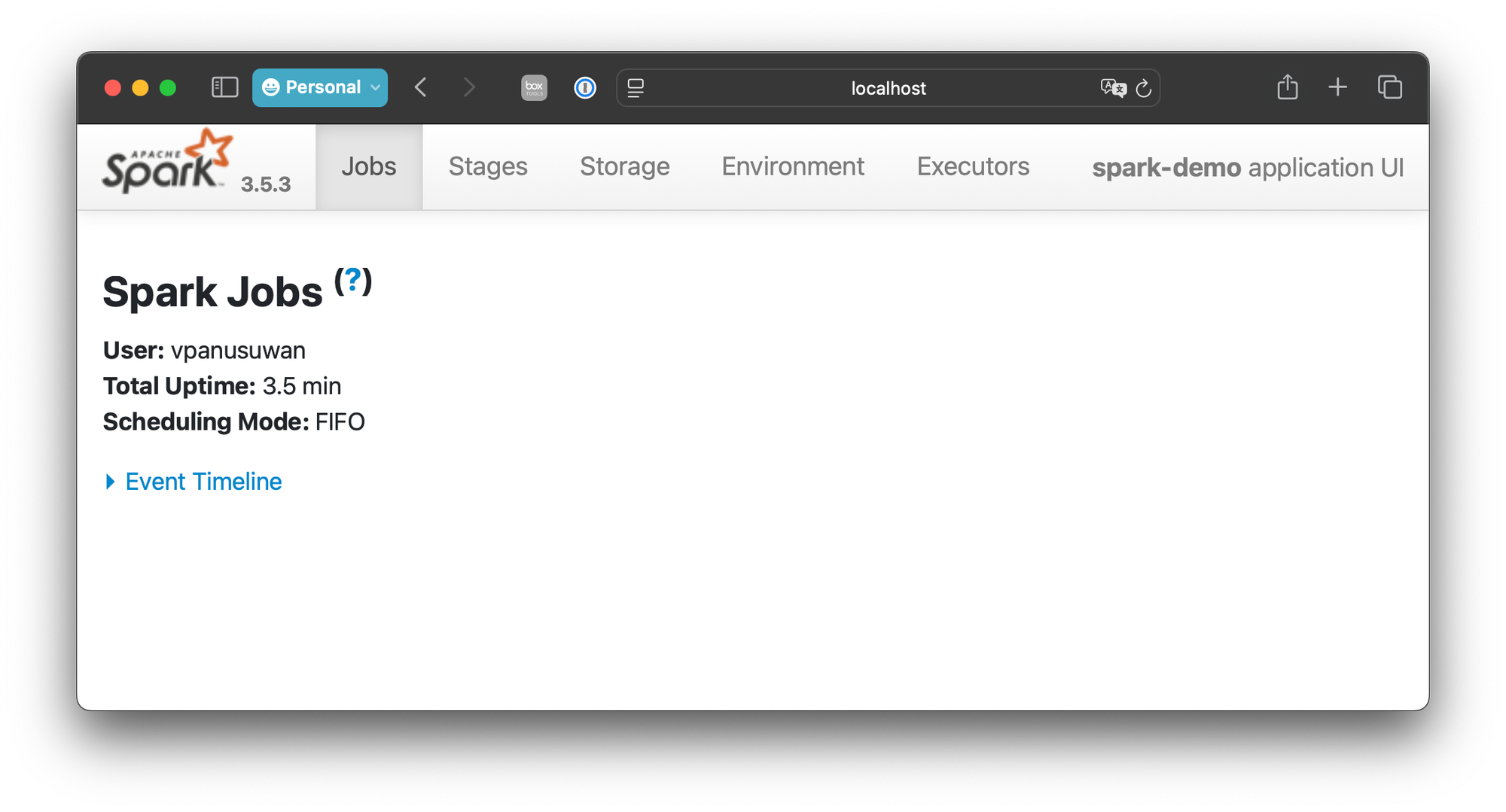
Local Iceberg Table
For a short experimentation with Local Iceberg table, we can configure sparks to store metadata in a local file. The example below shows how we could setup a catalog named local
, where the data files are stored at $PWD/warehouse
import os
cwd = os.getcwd()
spark = SparkSession.builder \
.master("local[*]") \
.appName("spark-demo") \
.config(map={
"spark.jars.packages": "org.apache.iceberg:iceberg-spark-runtime-3.5_2.12:1.7.0",
"spark.sql.extensions": "org.apache.iceberg.spark.extensions.IcebergSparkSessionExtensions",
"spark.sql.catalog.local": "org.apache.iceberg.spark.SparkSessionCatalog",
"spark.sql.catalog.local.type": "hive",
"spark.sql.catalog.local": "org.apache.iceberg.spark.SparkCatalog",
"spark.sql.catalog.local.type": "hadoop",
"spark.sql.catalog.local.warehouse": f"{cwd}/warehouse",
}) \
.getOrCreate()
after setting up spark session, we can create and use the iceberg tables from pyspark.
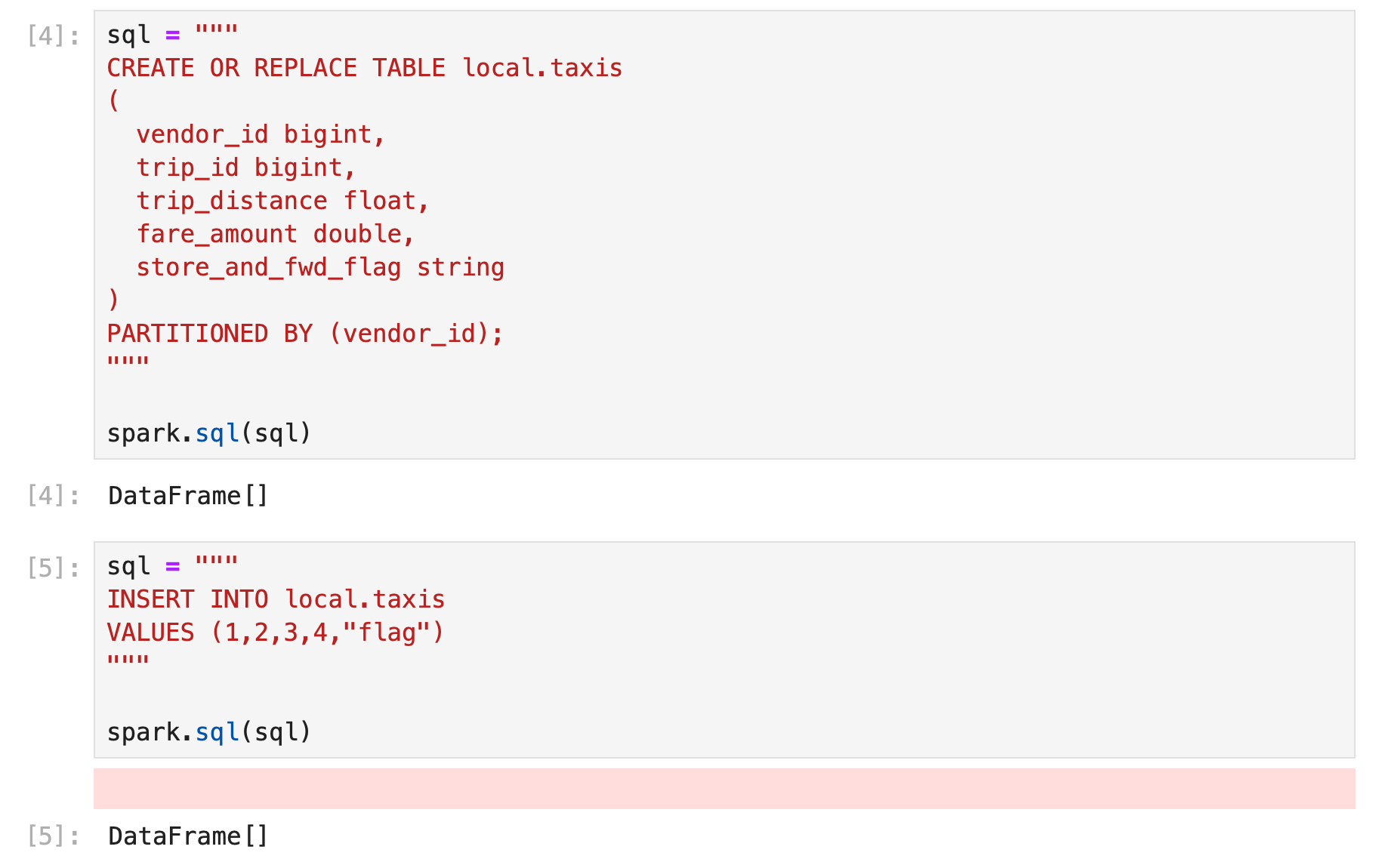
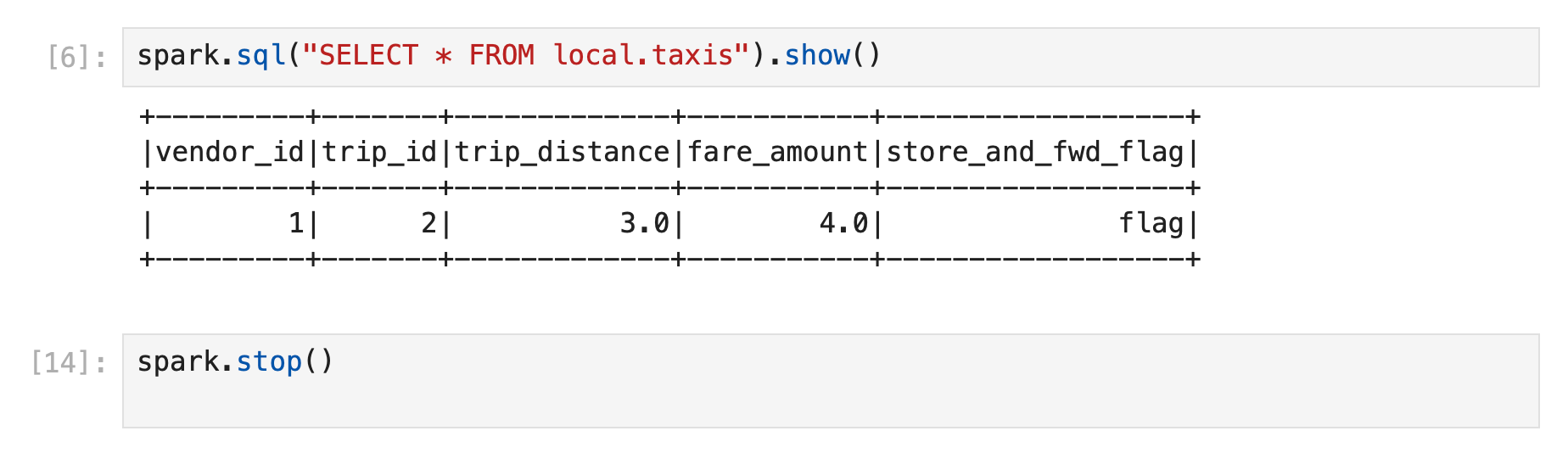
Data will be written to designated directory
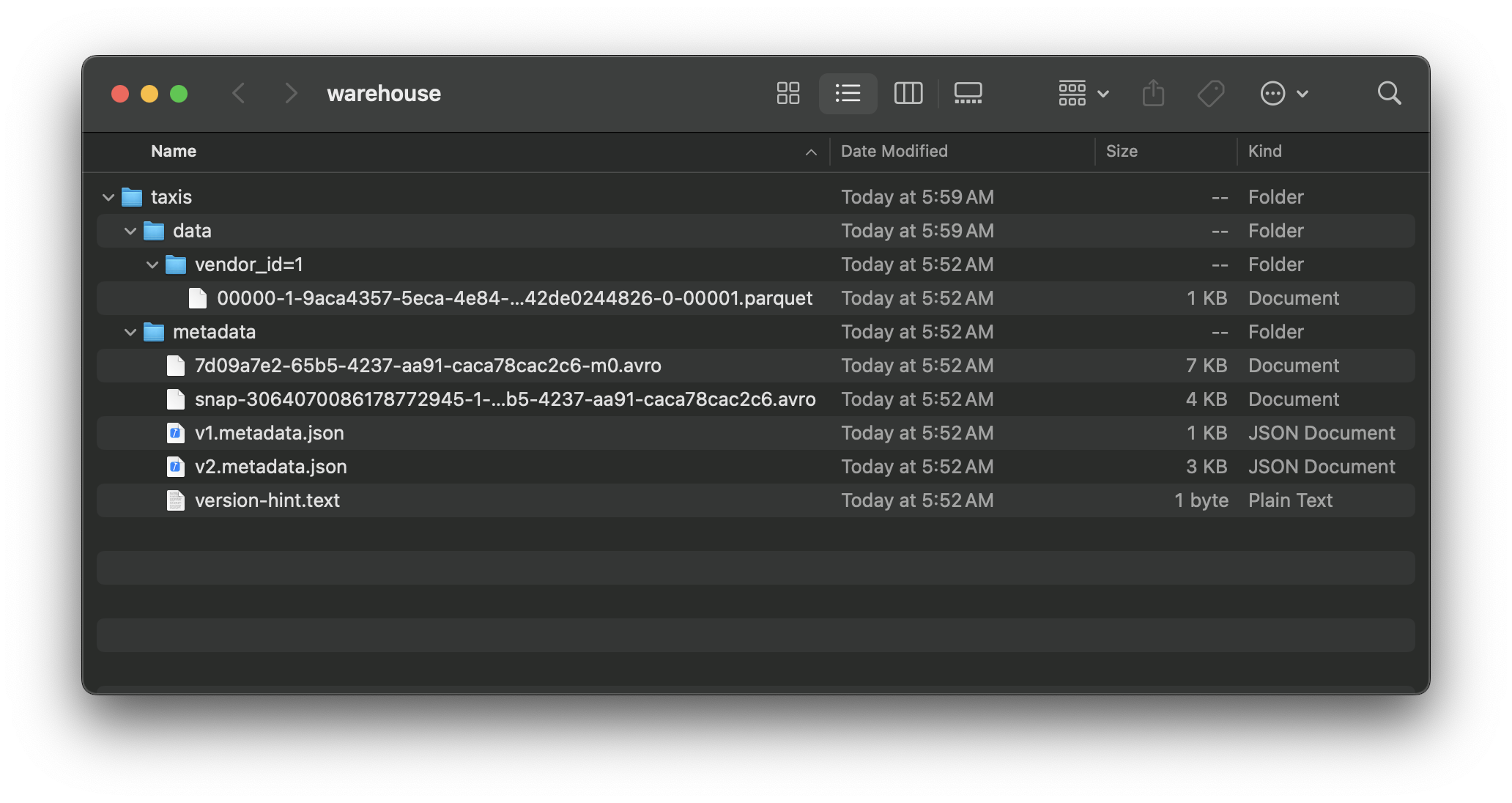